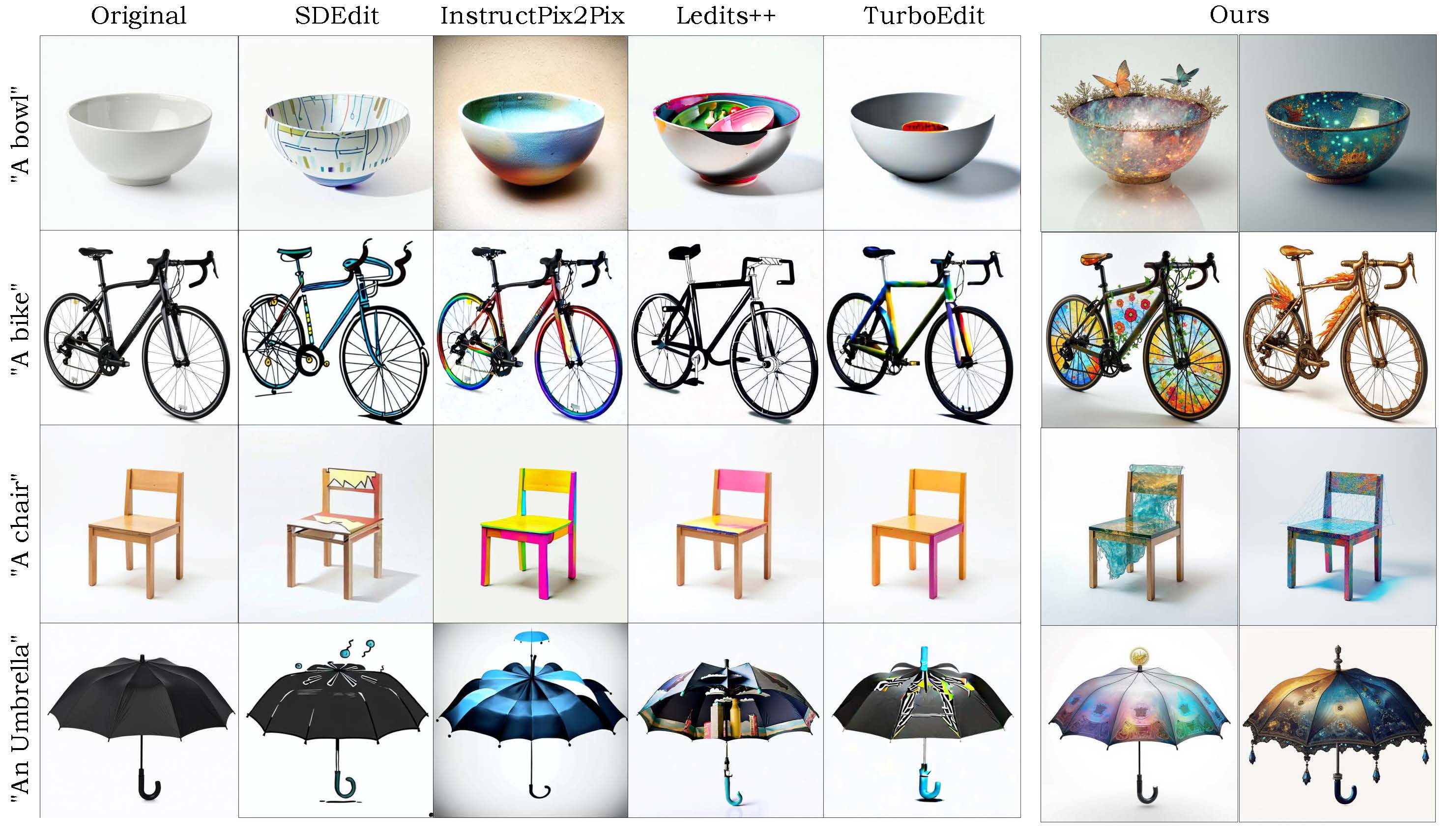
Creativity in AI-generated imagery remains a fundamental challenge, requiring not only the generation of visually compelling content but also the capacity to add novel, expressive, and artistically rich transformations to images. Unlike conventional editing tasks that rely on direct prompt-based modifications, creative image editing demands an autonomous, iterative approach that balances originality, coherence, and artistic intent. To address this, we introduce CREA, a novel multi-agent collaborative framework that mimics the human creative process. Our framework leverages a team of specialized AI agents who dynamically collaborate to conceptualize, generate, critique, and enhance images. Through extensive qualitative and quantitative evaluations, we demonstrate that CREA significantly outperforms state-of-the-art methods in diversity, semantic alignment, and creative transformation. By structuring creativity as a dynamic, agentic process, CREA redefines the intersection of AI and art, paving the way for autonomous AI-driven artistic exploration, generative design, and human-AI co-creation. To the best of our knowledge, CREA is the first work to introduce a disentangled approach and the first to employ an agentic framework for editing tasks.
Our method surpasses state-of-the-art methods across multiple metrics for both editing and generation tasks. Note that DINO scores cannot be computed for image generation, as they rely on image-image similarity, and there is no reference image available for this task. * indicates that scores are interpreted in opposition to their conventional usage as creative generation task benefits from greater perceptual distance between the original and edited images. Q1 measures generation usability, editing consistency while Q2 measures novelty/uniqueness.
Conventional metrics miss nuanced creative qualities. We therefore apply an LLM‑based judge (QWEN2.5-VL) to score key aspects of creativity, including Originality, Expressiveness, Aesthetic Appeal, Technical Execution, Unexpected Associations, Interpretability and Depth, and Overall Creativity, simulating human-like subjective assessments. CREA achieves the highest scores across all creativity dimensions, validating its superior creative performance.
@misc{venkatesh2025creacollaborativemultiagentframework,
title={CREA: A Collaborative Multi-Agent Framework for Creative Content Generation with Diffusion Models},
author={Kavana Venkatesh and Connor Dunlop and Pinar Yanardag},
year={2025},
eprint={2504.05306},
archivePrefix={arXiv},
primaryClass={cs.CV},
url={https://arxiv.org/abs/2504.05306},
}